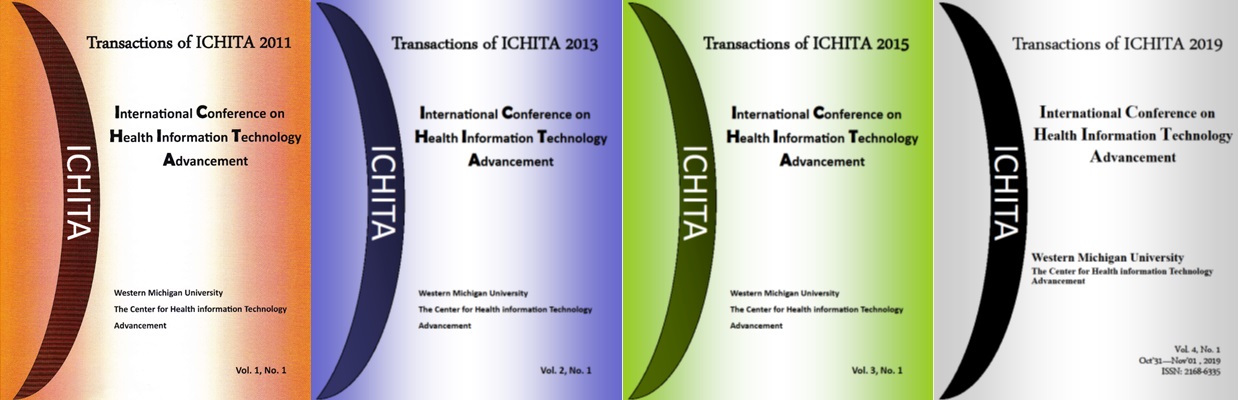
Transactions of the International Conference on Health Information Technology Advancement
Document Type
Article
Version
publisher_pdf
Publication Date
11-2019
Abstract
In many medical studies, an outcome of interest is not only whether an event occurred, but when an event occurred; and an example of this is Alzheimer’s disease (AD). Identifying patients with Mild Cognitive Impairment (MCI) who are likely to develop Alzheimer’s disease (AD) is highly important for AD treatment. Previous studies suggest that not all MCI patients will convert to AD. Massive amounts of data from longitudinal and extensive studies on thousands of Alzheimer’s patients have been generated. Building a computational model that can predict conversion form MCI to AD can be highly beneficial for early intervention and treatment planning for AD. This work presents a big data model that contains machine-learning techniques to determine the level of AD in a participant and predict the time of conversion to AD. The proposed framework considers one of the widely used screening assessment for detecting cognitive impairment called Montreal Cognitive Assessment (MoCA). MoCA data set was collected from different centers and integrated into our large data framework storage using a Hadoop Data File System (HDFS); the data was then analyzed using an Apache Spark framework. The accuracy of the proposed framework was compared with a semi-parametric Cox survival analysis model.
WMU ScholarWorks Citation
Alsaedi, Abdalrahman; Fong, Alvis; Abdelqader, Ikhlas; Delano, Mohammed Niaz; and Altaie, Khulud, "Modeling Big Medical Survival Data Using Decision Tree Analysis with Apache Spark" (2019). Transactions of the International Conference on Health Information Technology Advancement. 73.
https://scholarworks.wmich.edu/ichita_transactions/73